Ever wonder how a perfectly logical quality metric designed to save lives can spectacularly backfire? Let me tell you a true story about good intentions, data, and unintended consequences.
In 1997, JAMA published an article that stated that people who received antibiotics within 8 hours of arriving at the hospital had a higher chance of survival.1
This article sparked controversy and outrage, as hospitals were blamed for not appropriately treating people with pneumonia. In the early 2000s, CMS implemented a quality metric requiring antibiotics to be administered to people with pneumonia within 4 hours of arrival at the hospital—to save more lives. What could go wrong?
Under pressure to meet this timeline, Emergency department doctors began over-diagnosing pneumonia and administering unnecessary antibiotics to patients with any respiratory symptoms. Many patients with conditions like heart failure or viral infections received unnecessary antibiotics, leading to side effects and resulting in net harm. After widespread criticism from front-line physicians, CMS removed this metric.2
Welcome to the series on quality in healthcare, where we will discuss the quality of quality metrics. Over the next several articles (and videos), I will show you how the quest to improve quality, in reality, has nothing to do with improving patient care but everything to do with increasing profits.
This article is not a comprehensive guide to quality. My goal is to examine the paradox of healthcare quality measurement, i.e., how systems designed to improve care can sometimes achieve the opposite effect.
The video version of this article is embedded below and on my YouTube Channel.
Audio podcast and Video versions are also available on the Podcasts Page.
Defining Quality
Several definitions of quality have been proposed over the last century. The modern quality movement originated in manufacturing. Three of the earliest pioneers, William Shewhart, Joseph Juran, and Edward Deming, worked together at the Hawthorne Factory in the early 1900s and heavily influenced each other.3
The earliest definitions of quality are characterized by two dimensions:
“What the customer wants”: This subjective aspect recognizes that quality is ultimately defined by the user or consumer.
“What the product is”: This objective aspect focuses on the product's measurable characteristics and their conformance to specifications.
Phil Crosby4 took this definition one step further and redefined quality as:
Quality is conformance to requirements, Nothing more, nothing less.
Avis Donabedian extended these earlier concepts of quality, which were applied to manufacturing, to healthcare.5
The balance of health benefits and harm is the essential core of a definition of quality.
When Quality Meets Reality
Quality isn't just about how good something is, but also how well it fits the situation. Think about it this way: a winter coat is not useful in the summer, regardless of its quality. Without proper context, quality may be either useless, nuisance, or sometimes harmful, e.g., you don’t carry a warm, high-quality coat in summer (i.e., useless and nuisance), to being forced to wear the coat and suffer heat stroke.
Cynefin Framework
The Cynefin framework6 is a conceptual framework used to aid decision-making. It has five domains:
Clear: represents the “known knowns.” This means there are rules (or best practices) in place, the situation is stable, and the relationship between cause and effect is clear, i.e., if you do X, expect Y.
This framework recommends “sense-categorize-respond,” i.e., assessing the facts, categorizing them, and then applying the rule or best practice.
Complicated: represents the “known unknowns.” The relationship between cause and effect requires analysis or expertise, and there may be many right answers.
This framework recommends “sense–analyze–respond,” i.e., assess the facts, analyze them, and apply the appropriate good operating practice.
Complex: represents the “unknown unknowns.” Cause and effect can only be deduced retrospectively, and no good answers exist when the decision must be made.
This framework recommends “probe-sense-respond,” i.e., conducting small trials (probing the situation), sensing what works, and then responding appropriately.
Chaotic: also consists of “unknown unknowns,” with the added complexity of a rapidly changing situation, e.g., a doctor working in the ICU with several sick patients who are deteriorating rapidly.
Events in this domain are too confusing to wait for a knowledge-based response, and the recommended framework is “act-sense-respond.” Act first, assess if the action is working, i.e., sense, and then respond appropriately.
Confusion: This domain represents situations without clarity about which of the other domains apply.
When doctors determine treatment plans, they operate in domains that vary from complicated to chaotic depending on the number of medical problems, medications, and the acuity of sickness. If they cannot elicit meaningful medical history or obtain information, they operate in the domain of confusion. For example, even a “simple” case of upper respiratory infection (URI) would fall under the “complicated” domain. We take a good medical history (i.e., sense), analyze it to determine if the URI is more likely to be viral or bacterial, analyze the risks vs. benefits of antibiotics, and then respond with a treatment plan such as conservative management or antibiotics.
Also, remember the pneumonia quality metric. That was squarely in the “complex” domain. The cause-effect relationship between antibiotic administration within 4 hours of arriving at the hospital and survival rates was determined retrospectively, i.e., medical chart reviews after the event had already occurred.
Donabedian Triad
Now that we have defined quality and recognized the importance of context, let's explore the framework proposed by Donabedian to evaluate quality in healthcare.
Structure: evaluates whether there is adequate infrastructure, such as buildings, staff, and equipment, to deliver care.
Process: evaluates if all the necessary actions took place to ensure “appropriate” care.
Outcome: evaluates if the patient achieved the expected results, e.g., survival, complications, and/or quality of life.
Measuring outcomes is difficult, as outcomes generally take a long time to manifest. For example, measuring mortality may take decades. Therefore, most quality metrics are based on “processes.”
The Art & Science of “Appropriate Process”
Defining “appropriate process” is where the rubber hits the road. A process or plan is developed after the situation is “sensed” and “analyzed,” or, to put it in medical jargon, after a medical history and physical exam are performed, and either a differential diagnosis is formulated (for an acute medical problem), or several courses of action have been analyzed (for a chronic medical condition).
Let’s take two examples, one for an acute medical problem and the second for a chronic medical problem:
Antibiotics for Streptococcal Sore Throat
This measure requires that anyone diagnosed with “pharyngitis” or “tonsillitis” undergo a “strep test” within seven days of receiving an antibiotic.7 The goal is to decrease unnecessary antibiotic use, as most of these infections are viral. Under the “clear domain” in Cynefin framework, this translates to:
Sense: Medical history /Exam
Categorize: pharyngitis/tonsillitis or other cause of illness
Respond: Perform strep test, and if positive, prescribe antibiotics
This framework may apply to children and adults who don’t have other medical problems, but in people with multiple medical issues, this may fall under the “complicated” domain.
Sense: Medical history /Exam
Analyze: Does the person have pharyngitis/tonsillitis or also a secondary pneumonia infection?
Respond: The person is diagnosed with two concurrent medical issues: pharyngitis and pneumonia. A strep test is not performed, but antibiotics are prescribed for pneumonia.
In this case, a “national review committee” may make the tradeoff that there is a net benefit as this metric decreases unnecessary antibiotic use (which I agree with as long as people are not forced into high deductible health plans and have to pay for the strep test out of pocket!). However, this quality metric can potentially penalize doctors caring for very sick people.
Hemoglobin A1c Control for Patients with Diabetes
This measure requires controlling Hemoglobin A1c (HbA1c), a proxy measure for diabetes control, to be <8%. Under the “clear domain” in Cynefin framework, this translates to:
Sense: HbA1c is greater than 8%
Categorize: Diabetes is uncontrolled
Respond: Take action (e.g., prescribe medications) to reduce HbA1c < 8%.
However, poor control of HbA1c needs to be analyzed under the “complicated” and “complex” domains to take appropriate actions. For example:
Sense: HbA1c is greater than 8%
Analyze: Factors leading to HbA1c over 8%, such as the inability to afford medications, the tradeoff between limited life expectancy and quality of life (e.g., a person likes food), the risk of hypoglycemia (low blood sugar causing worse complications), or the person forgets to take medications.
Respond: Action may involve getting social workers involved to determine the best course of action at the risk of letting HbA1c be greater than 8%.
Quality metrics either assume that medical decisions are in the Cynefin framework's “clear” domain—or attempt to exclude medical nuances to recategorize them from the “complicated” and “complex” domains into the “clear” domain.
The act of defining an “appropriate process” that underlies quality metrics often transforms medical guidelines into rigid rules, i.e., doing X gets Y result.
Donabedian himself acknowledged this limitation when he defined quality in healthcare:
It is one part science and one part art.
In addition to defining the “appropriate process” that undergirds a quality metric, it must be standardized for reporting purposes.
While CMS does define some quality metrics, the main authority is the National Committee for Quality Assurance (NCQA). NCQA develops a common set of quality metrics with definitions called HEDIS (Healthcare Effectiveness Data and Information Set). Today, HEDIS measures serve as the universal language for assessing healthcare quality and are used by insurance companies, hospitals, and government agencies, including CMS.
Goodheart’s Law:
When a measure becomes a target, it ceases to be a good measure.
Or,
Any observed statistical regularity will tend to collapse once pressure is placed upon it for control purposes.
The challenge of simplifying complex medical decisions into clear metrics, combined with Goodhart's Law, creates a fundamental flaw in healthcare quality measurement.
The 4-hour pneumonia antibiotic metric—it led physicians to overdiagnose pneumonia and prescribe unnecessary antibiotics to anyone with respiratory symptoms—not to improve care but to meet the metric's requirements.
The strep throat testing metric, while intended to reduce unnecessary antibiotic use, can penalize physicians treating complex patients with multiple infections or encourage gaming the system by changing the medical diagnosis, for example, from pharyngitis to pneumonia.
Similarly, the HbA1c control metric for diabetes might incentivize physicians to either prescribe more medications that may lead to hypoglycemia or dismiss non-compliant patients from their practice. This will improve their quality scores rather than address the complex social and economic factors affecting diabetes control.
Additional Quality Challenge
How Metrics Compromise Informed Consent?
Quality metrics can fundamentally undermine the process of informed consent. When physicians are pressured to meet quality metric targets, they may either present treatment options that emphasize benefits that align with quality metrics while downplaying potential risks, or not discuss potential side effects of the recommended treatment option. For example, instead of having an open discussion about the pros and cons of tight diabetes control in elderly patients, a doctor might push for aggressive HbA1c management to meet the quality metric—despite an increased risk of hypoglycemia.
Therefore, quality metrics shift the doctor-patient relationship away from a collaborative decision-making process to meeting quality targets. This change overshadows the patient's right to make informed choices about their own care, which is the essence of informed consent.
The Evolution, or Erosion of Standard of Care
When physicians consistently present treatment options biased toward meeting quality metrics, patients begin to view these metric-driven decisions as the “standard of care.” This is reinforced by their discussions with family and friends who received similar care.
This shift creates a challenge for physicians. Those who wish to practice more nuanced, patient-centered medicine must now spend considerable time explaining why their recommended approach differs from what patients have come to expect. More concerning, if these physicians deviate from the metric-driven standard and their patient experiences a poor outcome, they face increased medicolegal vulnerability—even if their original medical decision was clinically sound and better suited to the patient's circumstances.
This alters the practice of medicine itself from a nuanced patient-centered approach (i.e., from complicated and complex Cynefin domains) to “cookbook medicine” (i.e., clear domain).
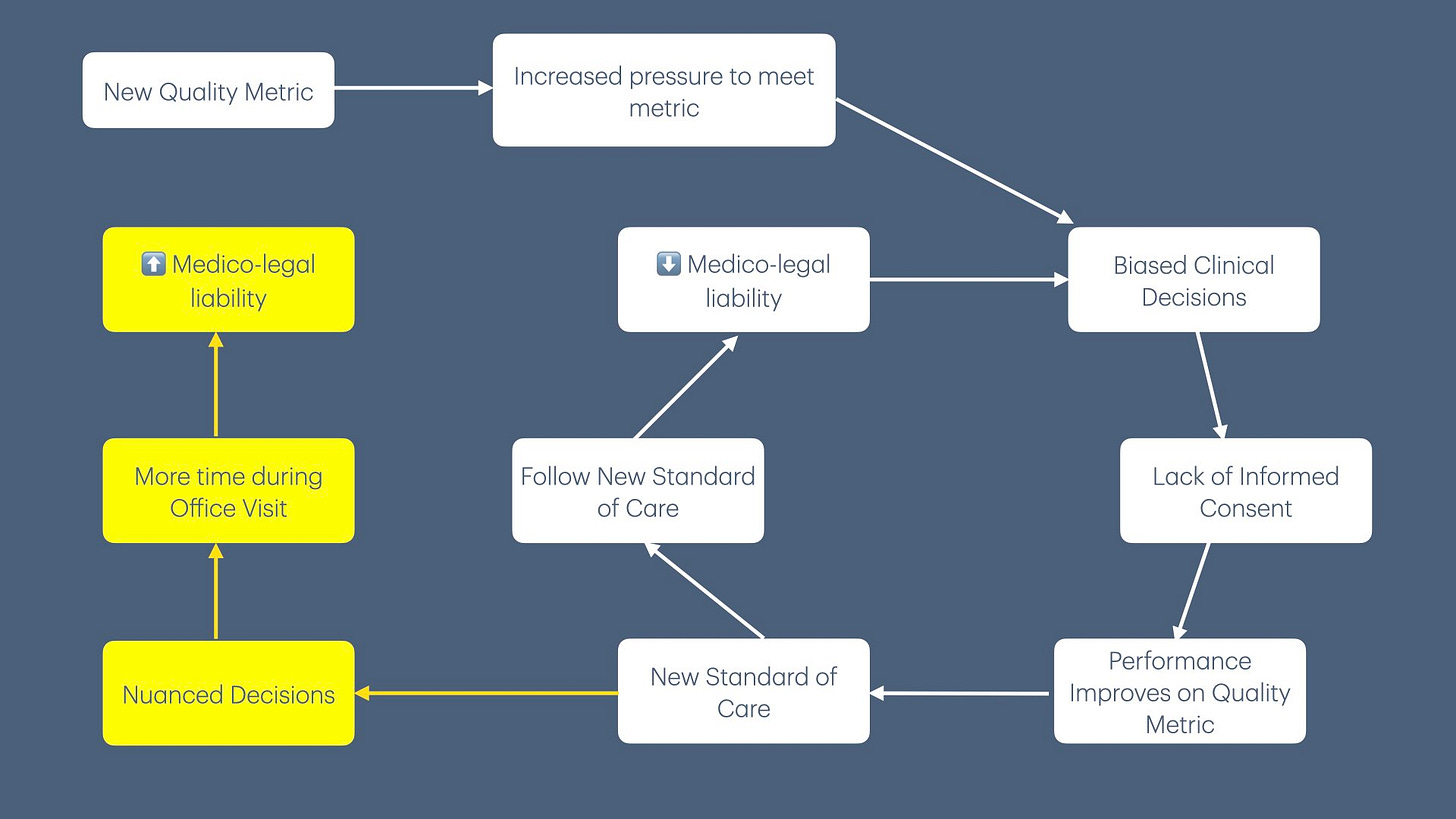
Mediocrity over Excellence
Quality metrics can create a leveling effect in healthcare. When metrics become the de facto standard of care, doctors often choose compliance over individualized patient care, effectively narrowing the performance gap by pulling top performers down while lifting lower performers up.
Increasing Complexity in the Pursuit of Quality
According to Charles Perrow’s theory of “Normal Accidents,”8 systems become more dangerous when they combine complexity (multiple interacting elements) with tight coupling (rigid, time-dependent processes). Healthcare quality metrics perfectly demonstrate this principle. When we attempt to simplify complex medical decisions into clear, measurable targets, we inadvertently create more rigid processes while adding new layers of complexity—exactly the combination Perrow warned against.
The pneumonia metric story illustrates this perfectly: a well-intentioned effort to standardize care through the 4-hour antibiotic rule made the system more tightly coupled (rigid timeframes, mandatory protocols), increasing complexity in a complex and chaotic emergency room environment. Rather than improving quality, this approach generated new risks, compromised clinical judgment, and harmed people.
Conclusion
Creating quality metrics requires balancing improved patient care against complexity at the point of care. While some metrics may justify their additional complexity (e.g., strep test for pharyngitis), most lack evidence from randomized controlled trials to support their implementation, and I have seen patient harm in real life from these metrics.
The question then is, why are we subjecting healthcare providers to this data collection exercise? The answer lies in Value-Based Contracts—these metrics have become a mechanism for increasing health insurance company profits.
Up Next
Now that we have explored some of the nuances of quality measures, the next article will delve into how and why quality measurement often functions more as a data collection and reporting exercise rather than a tool for genuinely improving quality.
If you liked this article, please consider sharing it.
Meehan, T. P., Fine, M. J., Krumholz, H. M., Scinto, J. D., Galusha, D. H., Mockalis, J. T., Weber, G. F., Petrillo, M. K., Houck, P. M., & Fine, J. M. (1997). Quality of care, process, and outcomes in elderly patients with pneumonia. JAMA, 278(23), 2080–2084.
Kanwar, M., Brar, N., Khatib, R., & Fakih, M. G. (2007). Misdiagnosis of Community-Acquired Pneumonia and Inappropriate Utilization of Antibiotics: Side Effects of the 4-h Antibiotic Administration Rule. Chest, 131(6), 1865–1869. https://doi.org/10.1378/chest.07-0164
Best, M., & Neuhauser, D. (2006). Walter A Shewhart, 1924, and the Hawthorne factory. Quality & Safety in Health Care, 15(2), 142. https://doi.org/10.1136/qshc.2006.018093
Crosby, P. B. (1979). Quality Is Free: The Art of Making Quality Certain: How to Manage Quality - So That It Becomes A Source of Profit for Your Business (First Edition). McGraw-Hill.
Brook, R. H., & Lohr, K. N. (1981). The Definition of Quality and Approaches to Its Assessment. Health Services Research, 16(2), 236. https://pmc.ncbi.nlm.nih.gov/articles/PMC1072233/
Mullan, F. (2001). A Founder of Quality Assessment Encounters A Troubled System Firsthand. Health Affairs, 20(1), 137–141. https://doi.org/10.1377/hlthaff.20.1.137
Snowden, D. J., & Boone, M. E. (2007). A leader’s framework for decision making. A leader’s framework for decision making. Harvard Business Review, 85(11), 68–76, 149.
Appropriate Testing for Pharyngitis. (n.d.). NCQA. Retrieved October 27, 2024, from https://www.ncqa.org/hedis/measures/appropriate-testing-for-pharyngitis/
Perrow, C. (1999). Normal Accidents: Living with High Risk Technologies - Updated Edition (REV-Revised). Princeton University Press. https://doi.org/10.2307/j.ctt7srgf
Hmm...I agree that poorly designed quality measures (antibiotics for pneumonia) are, on net, bad. I agree that all quality measures have negatives that need to be part of an cost/benefit evaluation. I agree that measures are probably net negative for the very best practitioners and systems. Incidentally, this is true for all metrics in all industries.
I don't think that it follows that all or most quality measures are bad on net, especially at the system/payer level. The A1c measure, for example, doesn't prescribe a treatment protocol (as the pneumonia one did.) Whether a patient's diabetes is uncontrolled due to lifestyle or medical factors, their health system (though maybe not an individual doctor) should try to help them improve it, and measuring success or failure is meaningful to incentivizing that.
I'm not totally clear on your argument yet though. One of the following?
1. All metrics are always or usually bad and healthcare quality metrics are no exception.
2. Quality measures in healthcare are always or usually bad for healthcare-specific reasons and should be eradicated.
3. Quality measures as they're currently implemented are bad, and they should be changed in xyz ways.
4. Quality measures are, on net, good, but not as uniformly beneficial as some VBC proponents think they are.